The ability to detect AI-generated content has become increasingly important in the digital age. As artificial intelligence systems continue to produce text that resembles human writing, distinguishing between machine-generated and human-created content is crucial for maintaining the authenticity and integrity of digital communication. With AI’s prevalence in content creation, recognizing the nuances of AI-written material is essential for journalists, readers, and content managers alike.
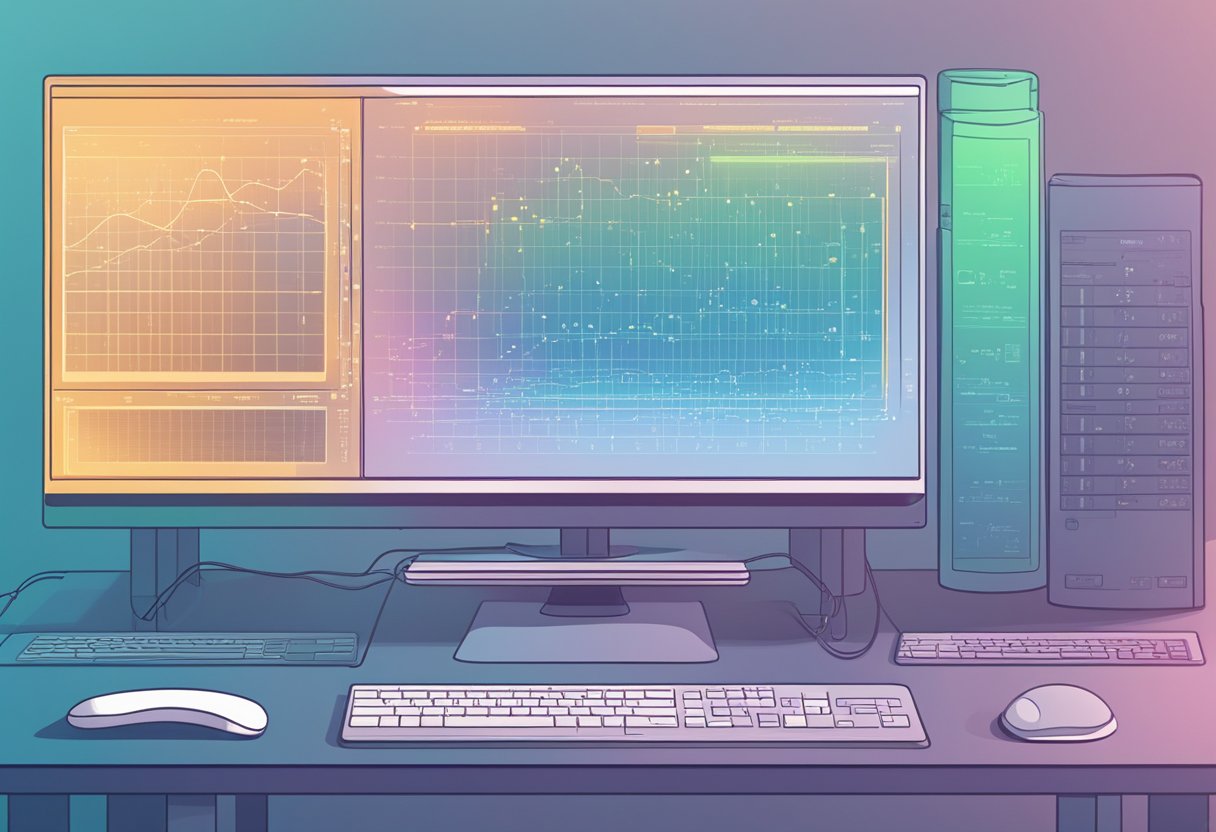
Identifying AI content involves discerning the subtle differences that reveal the absence of the human touch. AI-generated texts often lack the idiosyncratic nuances or contextual depth that human writers provide. There are proven methods for distinguishing human from machine writing that can help individuals detect AI involvement. These methods range from assessing the writing style and checking for overly standardized phrases to employing specialized tools designed to analyze text patterns.
Maintaining trust in media and the information ecosystem is paramount. Journalists and content creators strive to ensure that their work remains trustworthy and transparent. By implementing strategic AI detection and verification methods, individuals and organizations can future-proof their workflow and uphold the high standards expected in professional reporting and content creation. The integrity of human-authored content is preserved by cultivating skills in AI content detection, reinforcing the value of authentic human communication.
Understanding AI-Generated Content
AI-generated content is often characterized by its coherence, consistency, and adherence to a set of programmable patterns. To effectively identify such content, understanding the nuances of AI writing and how it differs from human writing is essential.
Characteristics of AI Writing
AI writing exhibits certain hallmarks that can be indicative of its non-human origin. These include:
- Repetitive Phrasing: A tendency to reuse certain phrases or syntactical structures.
- Lack of Nuance: AI may struggle with subtle nuances and the complex emotional undertones typically present in human writing.
- Consistent Style: Uniformity in tone and style, as AI maintains a consistent output according to its programming.
Identifying these characteristics requires careful reading and analysis and is an initial step in unveiling digital authorship.
Differences Between Human and AI Writers
When comparing AI-written content with that of humans, several differences become apparent:
Human Writers | AI Writers |
---|---|
Exhibit idiosyncrasies | Display uniform patterns |
Provide personal anecdotes | Lack personal experience |
Show variability in quality | Maintain a consistent quality |
Furthermore, human writers often bring a personal touch to their writing, something AI lacks. Recognizing these differences aids in discerning the origin of the content one encounters.
Technological Methods for Detection
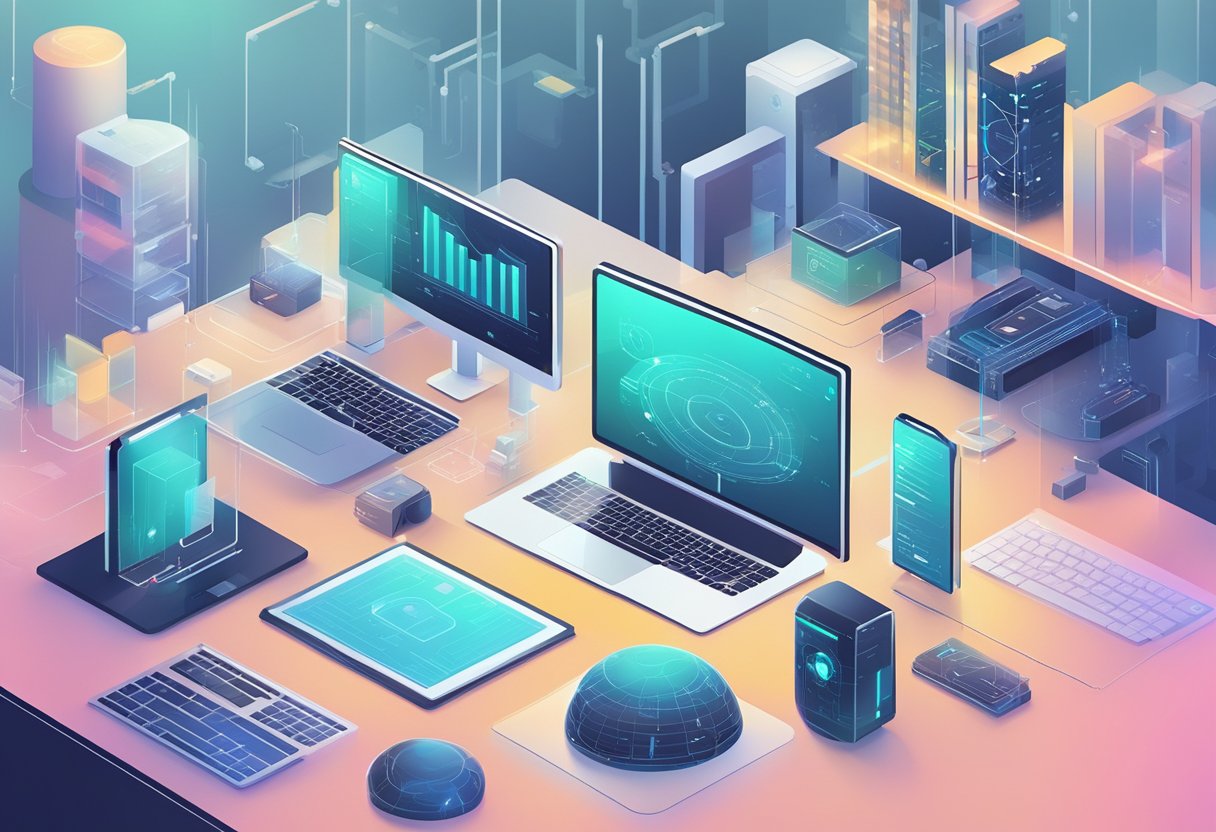
With advancements in technology, several tools and methods have been developed to identify AI-generated text effectively.
Machine Learning Classifiers
Machine learning classifiers can distinguish between human-written and AI-generated content by training on large datasets comprising both types of text. These classifiers use features such as grammar intricacies, sentence structure, and word choice to evaluate the likelihood of text being AI-produced. TensorFlow and scikit-learn are examples of libraries used to create custom detection models.
Natural Language Processing Tools
Natural language processing (NLP) tools analyze the structure and pattern of the text to detect AI fingerprints. By examining various linguistic features and inconsistencies, these tools, such as OpenAI’s API, provide a probability score indicating the chance of the content being machine-generated.
Stylometric Analysis Software
Stylometric analysis software evaluates the unique writing style of authors and detects deviations that suggest AI involvement. These programs assess aspects like sentence length, complexity, and vocabulary depth. Tools like JStylo and Anonymouth are utilized for such stylometric extrapolations.
Behavioral Approaches to Detection
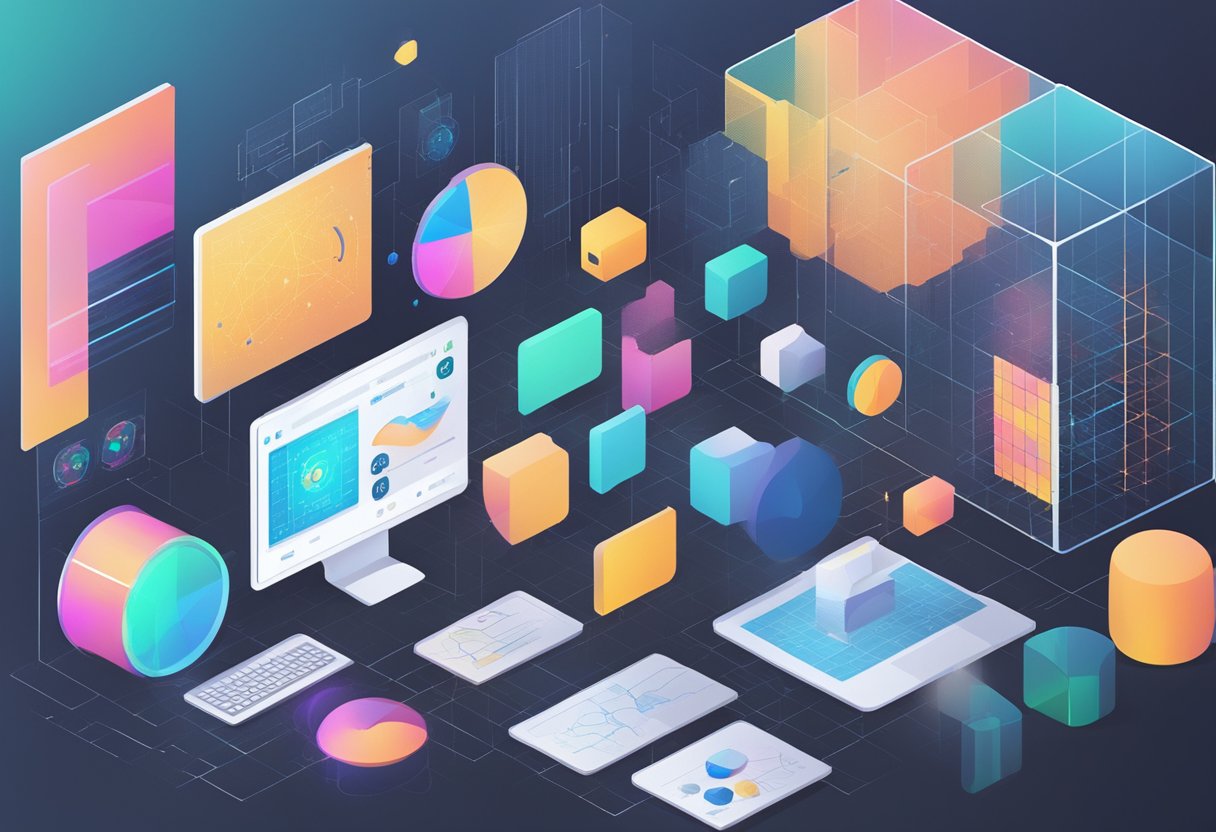
To detect AI-generated content, analysts can focus on interaction and usage patterns that are often distinctive for bots and automated systems.
Interaction Patterns Analysis
When examining Interaction Patterns, experts look for sequences that indicate non-human behavior. AI interactions tend to be:
- Predictable: AI responses are often uniform and may lack the variability typical of human behavior.
- Speedy: AI systems can generate responses at a pace that is usually unnaturally fast for a human.
Identifying these patterns involves:
- Timing analysis of responses.
- Assessment of conversational dynamics.
Usage Patterns Monitoring
Usage Patterns can be telling. They often display:
- Consistency: AI systems may operate 24/7 without breaks, whereas human activity typically shows cycles of availability.
- Volume: An AI might produce a higher volume of content than a human reasonably could over the same time period.
Monitoring includes:
- Analyzing login and activity timestamps.
- Tracking content output frequency.
These behaviors provide indicators that, when analyzed, can help distinguish between AI-generated content and human-originated interactions.
Legal and Ethical Considerations
In addressing the detection of AI content, one must consider the legal and ethical landscape that governs its creation and dissemination. Compliance with copyright laws and exploring the ramifications of undetected AI content are vital.
Copyright and AI-Generated Works
Copyright law protects original works of authorship. However, AI-generated content can complicate these protections. It raises questions such as:
- Who holds the copyright for AI-created works? The AI, the user, or the developer?
- How do copyright laws apply to derivative works created by AI?
The answers vary by jurisdiction and are subject to ongoing legal debate.
Ethical Implications of AI Content
The use of AI to create content carries several ethical implications. Key concerns include:
- Authenticity: Misrepresenting AI-generated content as human-created can be deceptive.
- Bias: AI may unintentionally perpetuate biases present in the data it was trained on.
Ethical use encourages transparency regarding the source of AI-generated content.
Regulatory Frameworks
Regulatory frameworks for AI are in their nascent stages, and they address aspects such as:
- Transparency:
- Regulations may require disclosure when content is AI-generated.
- Accountability:
- Identifying parties responsible for the output of AI systems.
Policies are being developed to ensure that AI-generated content adheres to standards that protect against misuse and uphold intellectual property rights.
Challenges and Limitations
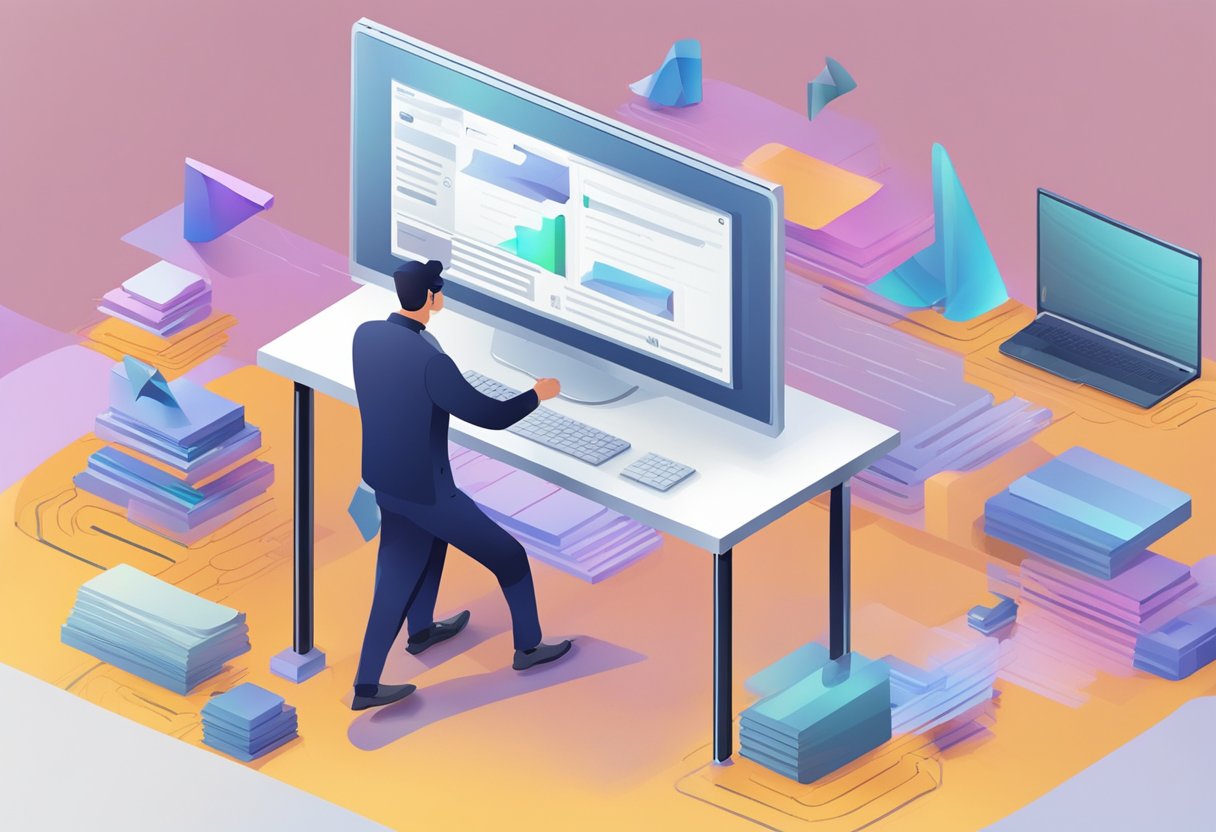
Detecting AI-generated content poses unique challenges and limitations due to the complexity and versatility of AI systems. These factors impact the efficacy and reliability of detection methods.
Evolving AI Capabilities
AI models are constantly improving, becoming more sophisticated at mimicking human writing styles. This rapid evolution means detection tools must continuously update to keep pace with new AI capabilities. They face the arduous task of distinguishing nuanced patterns that differentiate AI from human text, which is particularly difficult with state-of-the-art models that specialize in producing contextually rich and varied outputs.
False Positives and Negatives
The accuracy of AI content detection is not absolute; it yields false positives—identifying human content as AI-generated—and false negatives—failing to catch AI-authored text. The interplay between precision and recall in detection algorithms is crucial; high precision may increase false negatives, while emphasizing recall can cause false positives. Strategies to mitigate these errors include:
- Regularly updating detection models with diverse datasets to improve their discernment.
- Implementing multi-layered validation processes to reduce singular reliance on automated checks.
Scalability of Solutions
As the volume of digital content skyrockets, scalable detection solutions are vital. However, they must balance between being robust enough to handle large datasets and nimble enough to provide timely results. Solutions may require:
- Distributed computing to process large volumes of text.
- Machine learning optimizations to ensure models can be scaled without significant losses in accuracy or speed.
Future of AI Content Detection
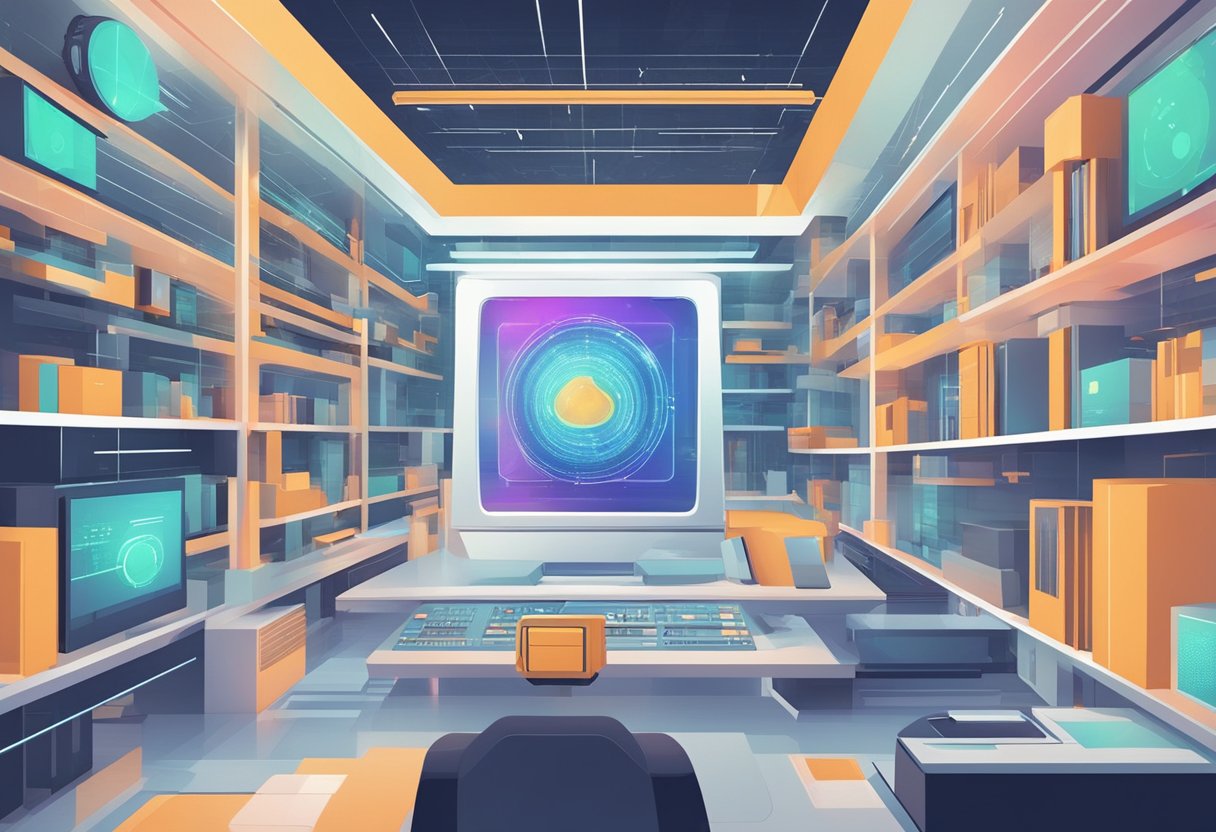
The landscape of AI content detection is rapidly evolving, with major advancements on the horizon in detection methods, ongoing research, and collaborative efforts that aim to standardize these processes.
Innovations in Detection Methods
Recent years have seen considerable progress in developing more sophisticated AI detection tools. Researchers are leveraging techniques like machine learning to better distinguish between content created by humans and AI. Future tools may incorporate behavioral analysis to assess patterns that are characteristic of AI-generated content. Additionally, there’s a growing trend towards deep learning models that can analyze text complexity and semantic coherence, providing more reliable detection capabilities.
Role of Ongoing Research
The field of AI content detection benefits significantly from ongoing academic and industry research. For example, studies that focus on the linguistic subtleties of AI-generated content are essential. Researchers are training AI to recognize subtle differences in syntax, word choice, and the logical flow of ideas. Moreover, advancements in natural language processing (NLP) algorithms play a crucial role as they improve the precision with which content can be analyzed for authenticity.
Collaborative Efforts and Standardization
There is a pressing need for collaboration between tech companies, academic institutions, and regulatory bodies to develop standardized methods for detecting AI-generated content. Such collaborations could lead to the creation of a global framework that ensures consistency in detection and regulation. The establishment of benchmarks and best practices, shared openly across industries, will help maintain the integrity of information and the reliability of content detection strategies.